Abstract
FUSE-MET addresses critical challenges in deploying human activity recognition (HAR) systems in uncontrolled environments by effectively managing noisy labels, sparse data, and undefined activity vocabularies. By integrating BERT-based word embeddings with domain-specific knowledge (i.e., MET values), FUSE-MET optimizes label merging, reducing label complexity and improving classification accuracy. Our approach outperforms the state-of-the-art techniques, including ChatGPT-4, by balancing semantic meaning and physical intensity.
Publication
The 39th Annual AAAI Conference on Artificial Intelligence (AAAI'25) - Student Abstract and Poster Program
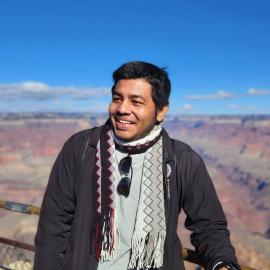
Graduate Research Associate
I am a Ph.D. student at Arizona State University. I work as a Graduate Research Associate at Embedded Machine Intelligence Lab (EMIL) under the supervision of Dr. Hassan Ghasemzadeh.
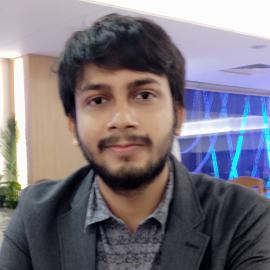
Graduate Research Associate
I am a Ph.D. student at Arizona State University. I work as a Graduate Research Associate at Embedded Machine Intelligence Lab (EMIL) under the supervision of Dr. Hassan Ghasemzadeh.
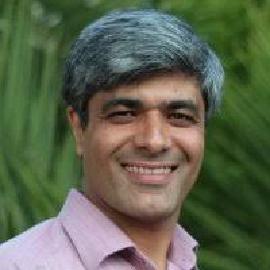
Director
Hassan Ghasemzadeh is an Associate Professor of Biomedical Informatics at Arizona State University (ASU) and a Computer Science Adjunct Faculty at Washington State University (WSU).