Edge AI
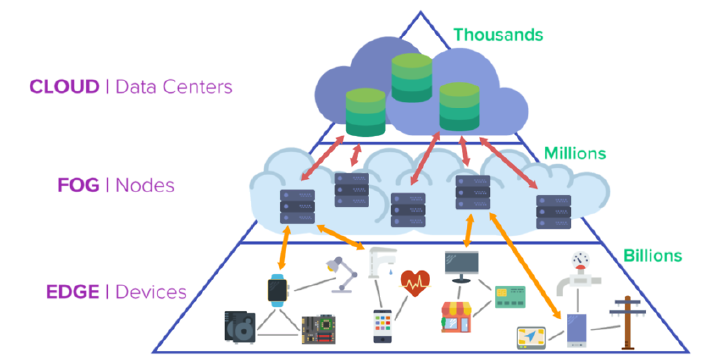
The stringent constrained resources available on tiny sensor nodes introduce a number of challenges regarding accuracy, power-efficiency, user-comfort, and security. These design considerations, however, often impose conflicting requirements. Thus, a comprehensive research approach to design future medical embedded systems and corresponding optimizations at different levels must consider these interdependent and conflicting requirements. We research methods of optimizing medical embedded systems for power-efficiency while taking into account other performance metrics. The goal is to develop tools, methodologies, and algorithms towards comprehensive approaches to address cross-layer optimization issues related to power, performance, user-comfort, and security.
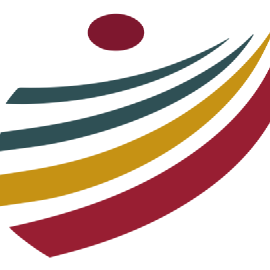
Embedded Machine Intelligence Lab
Research Lab
The current focus of our research in the Embedded Machine Intelligence Lab (EMIL) is on design, development, and validation of algorithms, tools, and technologies that enhance utilization and large-scale adoption of medical embedded systems. To validate and refine the new technology, we conduct clinical studies involving patients with heart failure, diabetes, cancer, visual impairment, and gait difficulties. Clinical studies are conducted in collaboration with partners from Elson S. Floyd College of Medicine, College of Nursing, College of Pharmacy, College of Education, and College of Agricultural, Human, and Natural Resource Science at WSU as well as our collaborators at Pullman Regional Hospital, UCLA School of Medicine, UCLA Stein Eye Institute, UC-Irvine Nursing Science, and Memorial Sloan Kettering Cancer Center (MSKCC). This end-to-end approach results in innovative, evidence-based and cost-conscious solutions for patients, doctors and medical centers.